Mobile app advertising: How machine learning turns data into customers
With the rapid growth of programmatic advertising in the mobile app space, discover how machine learning is enhancing ad targeting and performance in this Q&A with Andry Supian (head of product, Liftoff).
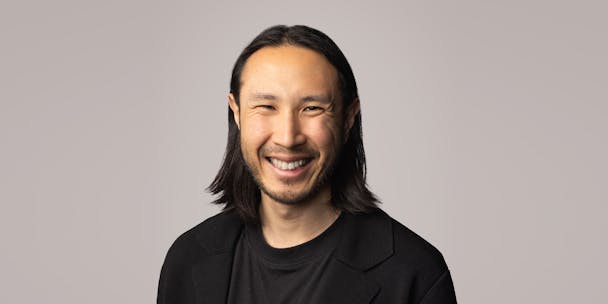
Most online ads are now transacted programmatically. Over the last 10 years, global spend on online programmatic advertising grew to nearly 70% of the entire pie (from 10%), and mobile ad spend reached $362bn last year.
In this Q&A, Andry Supian, head of product at Liftoff, explains the challenges surrounding the immense volume of ad opportunities in the mobile app programmatic space, and how machine learning can solve one of advertising’s biggest challenges: sifting through immense volume to find life-long customers.
Despite the widespread adoption, advertisers still struggle with making programmatic channels performant. Why do you think that is?
Three reasons: volume, variety, and velocity. At Liftoff, we see several billion users monthly across hundreds of thousands of mobile apps. Daily, this creates over 640 billion ad impression opportunities from ad exchanges. These apps use a variety of set-ups to monetize, which makes the pathways to reach these end users overwhelming and sometimes duplicative. As someone looking to market your game or an e-commerce app to this audience, figuring out where to start is daunting. Determining economic pathways at scale is also a very complex problem to solve.
Explore frequently asked questions
What is machine learning and how can it be applied to mobile app advertising to solve this challenge?
Although used for quite some time, machine learning (ML) has been the topic du jour in the technology landscape over the last two plus years. In app advertising, ML is designed to take different datasets from apps and from the marketers who buy advertising in that medium and use them to determine where and when to serve ads.
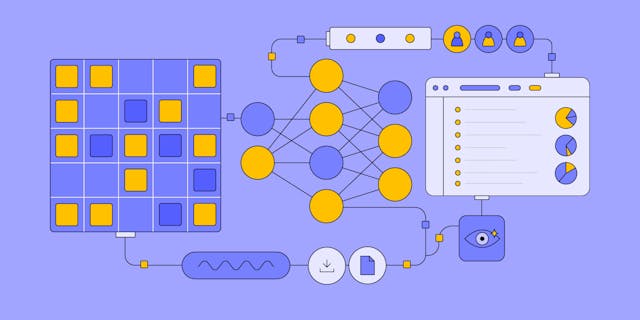
Machine learning is an effective way for advertisers to promote their apps through the programmatic channel because of the self-learning that takes place. ML derives useful patterns from large sources of information in ways that exceed the capabilities of humans. It can use its prior knowledge to help advertisers pick the right starting point for the campaign: users that are most likely to be interested in the product. From there, it can iterate based on observed outcomes. ML repeatedly shows the computer what “good” and “bad” look like over and over. This is where the “self-learning” happens. It’s impossible to replicate this manually at the speed and scale required to make programmatic advertising work.
How does ML change the overall advertising landscape?
Traditionally, marketers would try to dictate ad buying behavior based on audience segments, usually sourced from third-party data. What’s different with machine learning, especially in the app space, is it doesn’t preemptively determine who that audience is going to be. It lets the machines make an association between the outcome the marketer wants, and the user who's going to receive an ad. With machine learning, we no longer need to specify the inputs (like manually curated publisher lists or defined price points). Instead we need to specify goals (“look for users that completed a form”).
Advertisement
What helps make machine learning successful in mobile app advertising?
ML is an effective technology to sift through the high volume of users and bid opportunities, and it doesn’t have to be unconstrained. We can layer guardrails (blocklists, suppression lists, custom rules) to allow campaigns to benefit from the knowledge the advertiser already has on top of ML. However, the data has to be truthful. Truthfulness is more than cleanliness. The data needs to accurately represent the environment that marketers want the model to learn from.
Think about a model as a representation of something, like a mental model or a business model. ML models in the app ads ecosystem are no different. They’re a mathematical representation of the data it sees. So the data has to be clean, current and as descriptive as possible about the climate in that environment.
If you're working directly or indirectly with ML in advertising, what are some things you can do to set you up for success?
An important ML application is creative strategy. Machine learning can identify valuable pattern recognition to determine the optimal creative messaging. But remember at the end of the day, an individual user is seeing an ad creative, which will elicit feelings and cause them to take action. Think of ML as another trusted colleague in the room. It will amplify your intellect but won't replace human creativity and teamwork.
Content by The Drum Network member:

Liftoff
Liftoff helps mobile businesses maximize their revenue. It provides machine learning-powered marketing, monetization, and creative solutions that create better ad...
Find out more